
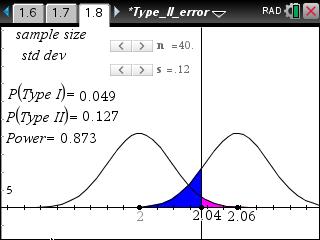
There are several ways to reduce the risk of making a type 1 error: For example, if a new drug is tested and found to be effective but later discovered that it actually causes more harm than good, this would be a type 1 error. Type 1 errors can have serious consequences, especially in the field of medicine or criminal justice. This means that there is evidence to support the alternative hypothesis when in reality, there is none. Type 1 errors, also known as false positives, can occur when a test or experiment rejects the null hypothesis incorrectly. It can help you avoid making incorrect decisions and ensure accurate research studies. Overall, it's essential to have a good understanding of type 1 errors.

Otherwise, their results could be skewed. When designing studies and analyzing data, researchers need to account for the possibility of false positives. Type 1 error is also important to understand from a statistical standpoint. This is especially important when the consequences of a wrong decision could be serious. If you know that there's a chance of a false positive, you can be more cautious in your interpretation of results. It's important to understand type 1 errors because it can help you avoid making decisions based on incorrect information. Why Is It Important to Understand Type 1 Errors The level of significance, sample size, power of the test, and the Bonferroni correction are all important considerations when trying to avoid a type 1 error. The higher the power of the test, the less likely it is that a type 1 error will occur.įinally, if there are multiple tests being conducted, the Bonferroni correction can be used to control for the possibility of a type 1 error.Īll of these factors contribute to the likelihood of a type 1 error. Third, the power of the test also affects the likelihood of a type 1 error. The larger the sample size, the less likely it is that a type 1 error will occur.

Second, the sample size also plays a role in the likelihood of a type 1 error. The higher the alpha level, the more likely it is that a type 1 error will occur. There are several factors that can contribute to a type 1 error.įirst, the researcher sets the level of significance (alpha). However, it's not always possible to completely eliminate these errors, so it's important to be aware of their potential impact when interpreting test results. Type 1 and Type 2 errors can be reduced by using more reliable tests and increasing the sample size. This error can have just as serious consequences as a Type 1 error, so it's important to be aware of both when interpreting test results. It's also worth considering the Type 2 error, which is when a test incorrectly indicates that a condition is not present when it actually is. On the other hand, if the consequences of a false positive are not so serious, then a lower level of proof may be acceptable. If the consequences of a false positive are serious, then a higher level of proof may be needed to make sure that the results are accurate. When interpreting results from a test, it's important to consider the potential for Type 1 errors. It's important to consider these factors when designing a study, as they can greatly impact the results. For example, if the sample size is too small or there is bias in the selection of participants, this can increase the likelihood of a Type 1 error. Type 1 errors are often due to chance, but they can also be caused by errors in the testing process itself. This error can have serious consequences, as patients may be needlessly exposed to harmful side effects or may miss out on treatment altogether. A Type 1 error, also known as a false positive, is when a test incorrectly indicates that a condition is present when it is not.įor example, if a new drug is tested and the null hypothesis is that the drug is ineffective but actually effective, then a Type 1 error has occurred.
